As artificial intelligence (AI) becomes more intricately woven into our daily lives, questions about transparency and trust in AI systems are growing louder. Enter XAI770K—a concept and model framework grounded in the principles of Explainable AI (XAI). The idea isn’t just to build intelligent systems, but to create ones that can explain their decisions to humans in a way that’s understandable and trustworthy.
Contents
What is XAI770K?
XAI770K represents a suite of algorithmic tools and design philosophies meant to enhance transparency in AI systems. At its core, it’s about making AI decisions interpretable to humans, even in highly complex models such as deep learning neural networks.
The “770K” in the name doesn’t refer to any standard measurement. Instead, it symbolizes the vast scope of use cases—over 770,000 scenarios—where explainability is vital. Whether it’s healthcare, finance, transportation, or legal tech, the demand for AI that can explain itself is rapidly rising.
Why Explainability Matters
As AI takes on an increasing role in decision-making processes, from loan approvals to medical diagnoses, understanding why a model made a specific decision isn’t just desirable—it’s essential.
- Accountability: Stakeholders can hold AI systems accountable if their workings are transparent.
- Bias Detection: Hidden biases in training data can be revealed through better model interpretability.
- Legal Compliance: Regulations in sectors like finance require a rational explanation for decisions.
- User Trust: Users are more likely to embrace AI if they understand how it works.
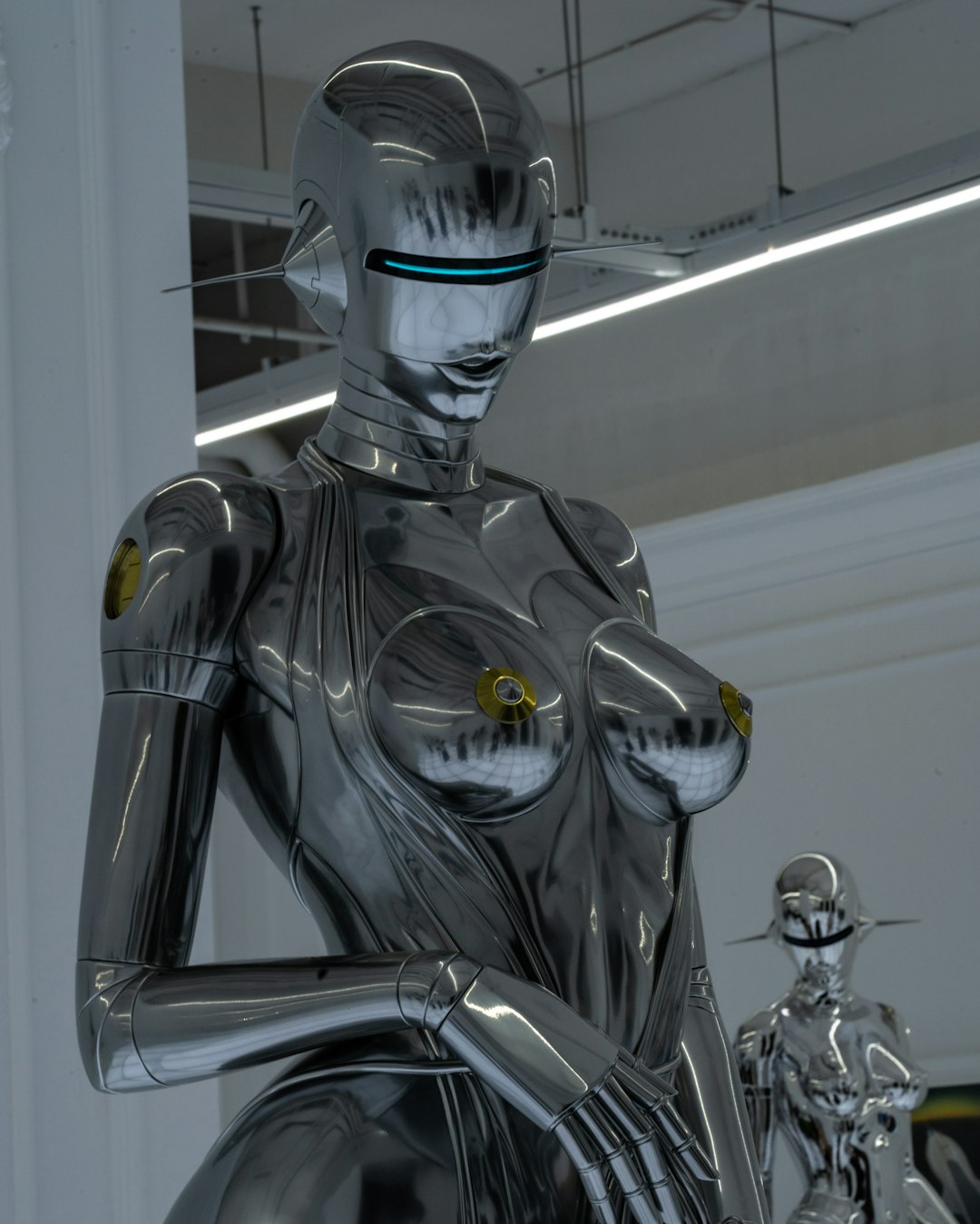
How XAI770K Works
XAI770K isn’t a single algorithm but rather a framework integrating several explainability techniques such as:
- LIME (Local Interpretable Model-Agnostic Explanations): Highlights which features influenced a specific prediction.
- SHAP (SHapley Additive exPlanations): Calculates the contribution of each feature to a prediction.
- Counterfactual Explanations: Demonstrates how input features would need to change to yield a different outcome.
By combining these with advanced visualization tools, XAI770K enables domain-specific customization—an AI used in oncology, for instance, can be tailored to explain predictions to medical professionals clearly and concisely.
Applications Across Industries
The reach of XAI770K extends across multiple domains:
- Healthcare: Doctors use AI to assist in diagnosing conditions. XAI helps validate the correctness and fairness of those suggestions.
- Finance: Banks rely on scoring algorithms; XAI can ensure decisions are fair and not discriminatory.
- Criminal Justice: Predictive policing and sentencing tools face scrutiny, and explainability is crucial to legitimacy.
- Autonomous Vehicles: Car manufacturers use XAI to understand failures or decisions made by self-driving systems.
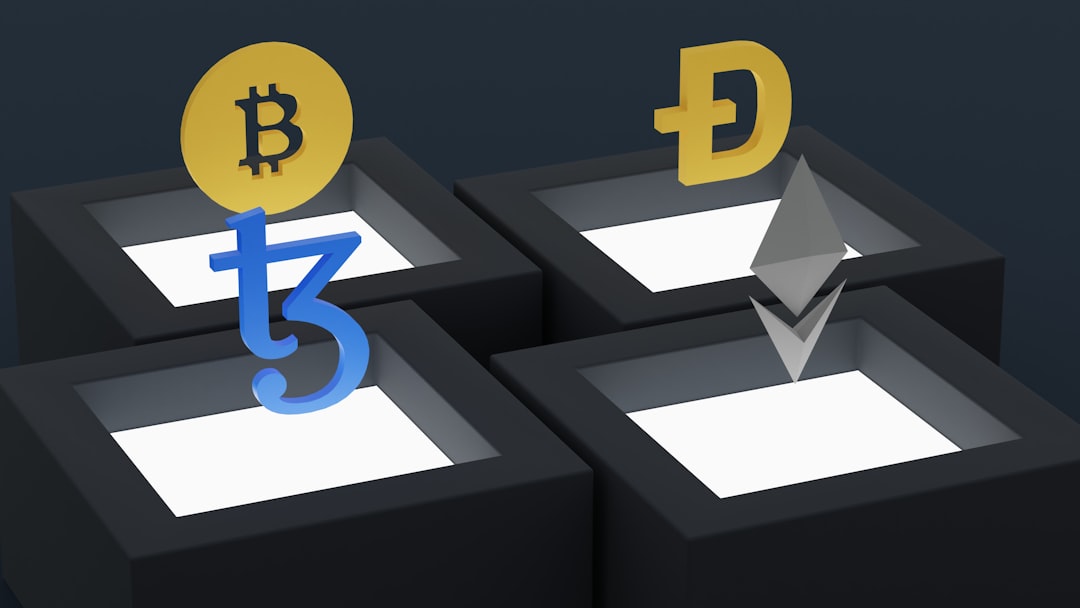
The Challenges Ahead
While the goals of XAI770K are clear, implementation poses significant hurdles:
- Complexity of Models: Deep learning models are often called “black boxes” for a reason; mapping their logic to human-understandable narratives is no simple feat.
- Trade-off Between Accuracy and Interpretability: Simpler models are more interpretable but may lack the predictive power of complex ones.
- Standardization: Without industry-wide benchmarks, it’s challenging to declare an AI system as “explainable.”
Despite these challenges, research and collaboration are moving the field forward. Open-source libraries like Google’s What-If Tool or DARPA’s XAI program are paving the way for more intuitive and accountable AI systems.
Looking Forward
As regulatory bodies and public sentiment push for greater transparency in artificial intelligence, models like XAI770K could become the gold standard. It’s not just about teaching machines to think—it’s about ensuring they can communicate their reasoning effectively.
By embracing XAI770K, organizations are not only investing in smarter technology but also cultivating a culture of ethics, responsibility, and trust—values that modern AI cannot afford to ignore.